MCP servers, part of the Model Context Protocol (MCP) introduced by Anthropic in November 2024, are programs that enable AI models, like large language models (LLMs), to connect with external data sources and tools in a standardized, secure way. Think of them as universal adapters—often likened to a “USB-C for AI”—that allow AI to interact with databases, APIs, file systems, or services like Google Drive, Slack, or GitHub without needing custom integrations for each.
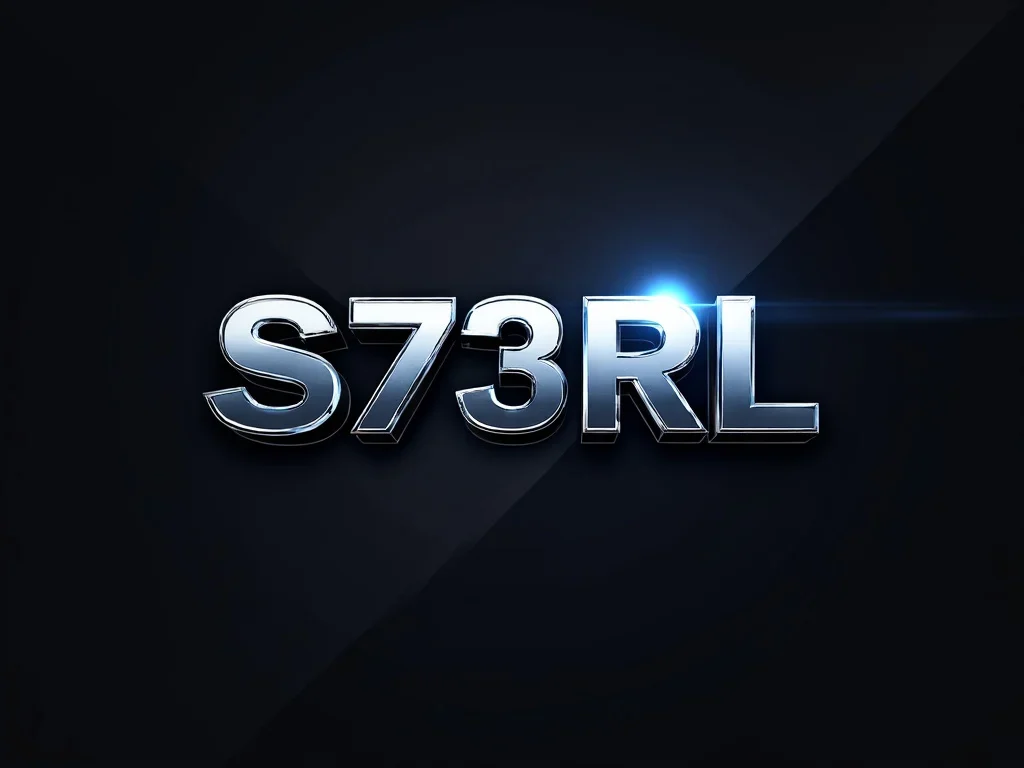

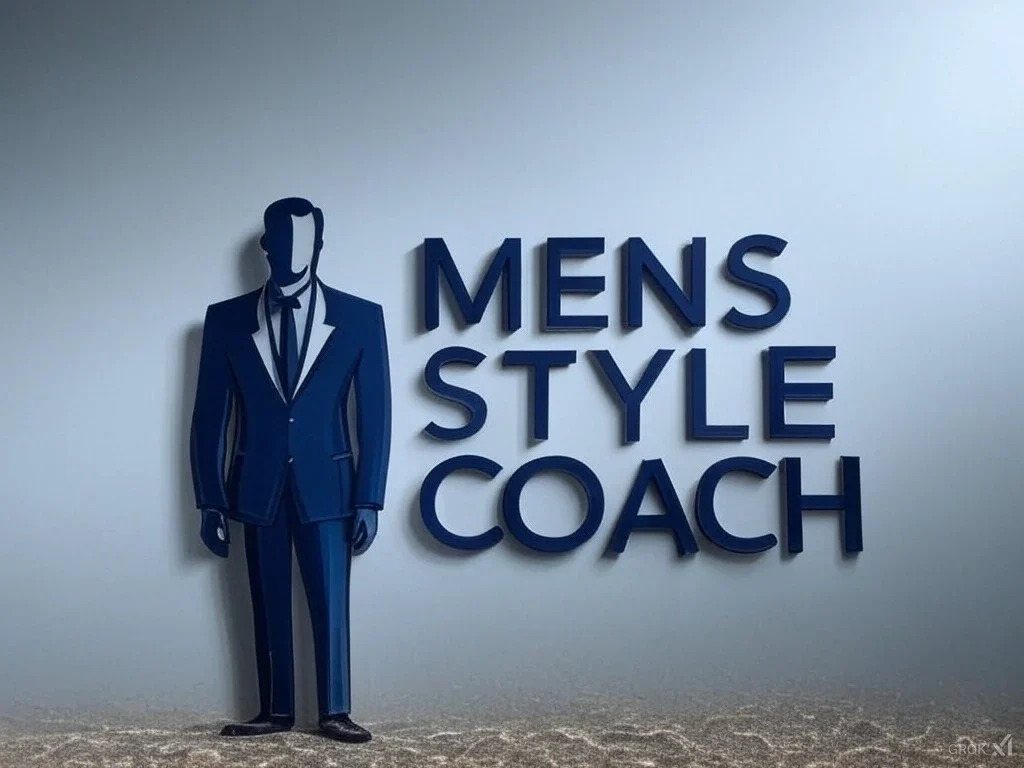
Key Points:
- Function: MCP servers act as bridges, exposing tools, resources, or prompts to AI applications. They let AI fetch real-time data (e.g., documents, database queries) or perform actions (e.g., create a GitHub issue) by providing a common interface.
- Architecture: MCP follows a client-server model:
- MCP Hosts: AI apps (e.g., Claude Desktop, IDEs) that use LLMs.
- MCP Clients: Manage secure, one-to-one connections between hosts and servers.
- MCP Servers: Lightweight programs that connect to local (e.g., files) or remote (e.g., APIs) data sources.
- Capabilities: They provide:
- Resources: Data like files or database records.
- Tools: Functions for actions (e.g., API calls).
- Prompts: Templates for consistent AI interactions.
- Benefits:
- Standardization: Any AI model (Claude, GPT-4, open-source LLMs) can use MCP servers, reducing the need for custom code.
- Security: Credentials stay server-side, and user approval is often required for actions.
- Scalability: Over 1,000 community-built servers exist as of February 2025, covering tools like Notion, Spotify, and even Blender for 3D modeling.
- Standardization: Any AI model (Claude, GPT-4, open-source LLMs) can use MCP servers, reducing the need for custom code.
- Use Cases:
- Enterprise: Connecting AI to internal tools like Jira or Confluence.
- Development: AI co-pilots managing code repositories via GitHub or Git servers.
- Daily Tasks: Automating file management, Slack communication, or location-based tasks with Google Maps.
- Enterprise: Connecting AI to internal tools like Jira or Confluence.
- Challenges:
- Managing multiple servers can be complex, especially in cloud or multi-user setups.
- Some users report setup difficulties or bugs, and security concerns arise when handling sensitive data like database credentials.
- Adoption is strong within Anthropic’s ecosystem (e.g., Claude), but broader support across other AI platforms is still growing.
- Managing multiple servers can be complex, especially in cloud or multi-user setups.
Why It Matters:
MCP servers address AI’s isolation from real-time data, unlike traditional systems like Retrieval-Augmented Generation (RAG), which rely on pre-indexed data. They enable dynamic, context-aware AI interactions with lower overhead and better security, potentially transforming how AI integrates into workflows.
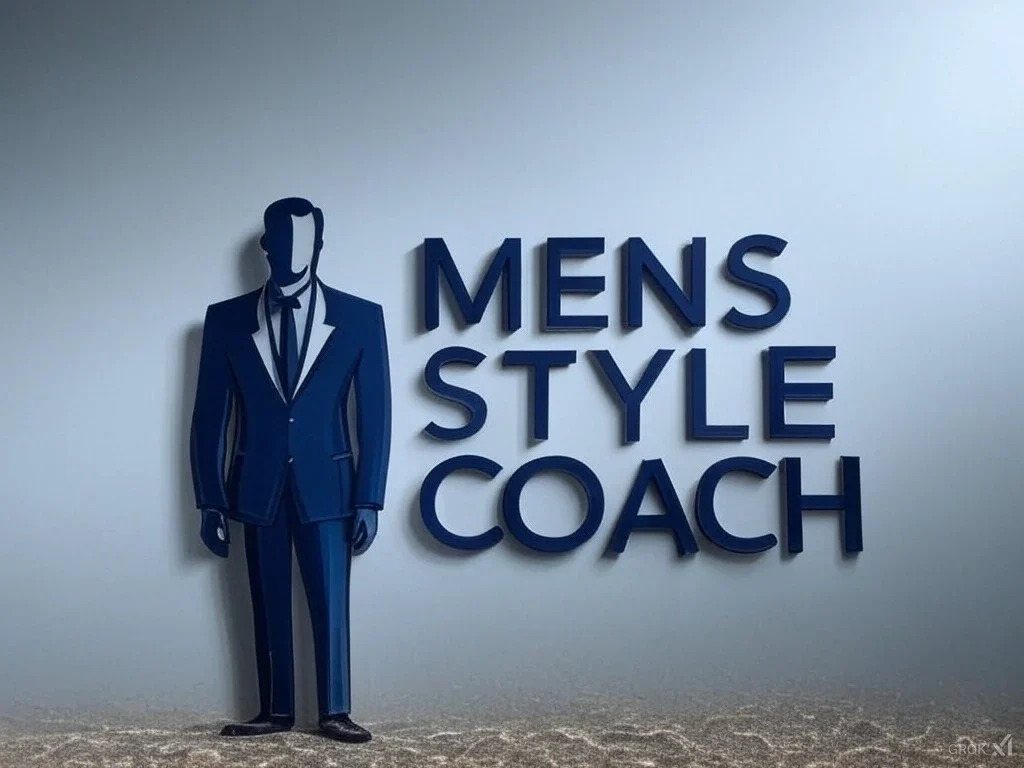

Getting Started:
Developers can build MCP servers using SDKs in Python, TypeScript, Java, or Rust, or use pre-built servers from modelcontextprotocol.io. For example, setting up a file system server involves adding a JSON config to an AI client like Claude Desktop.
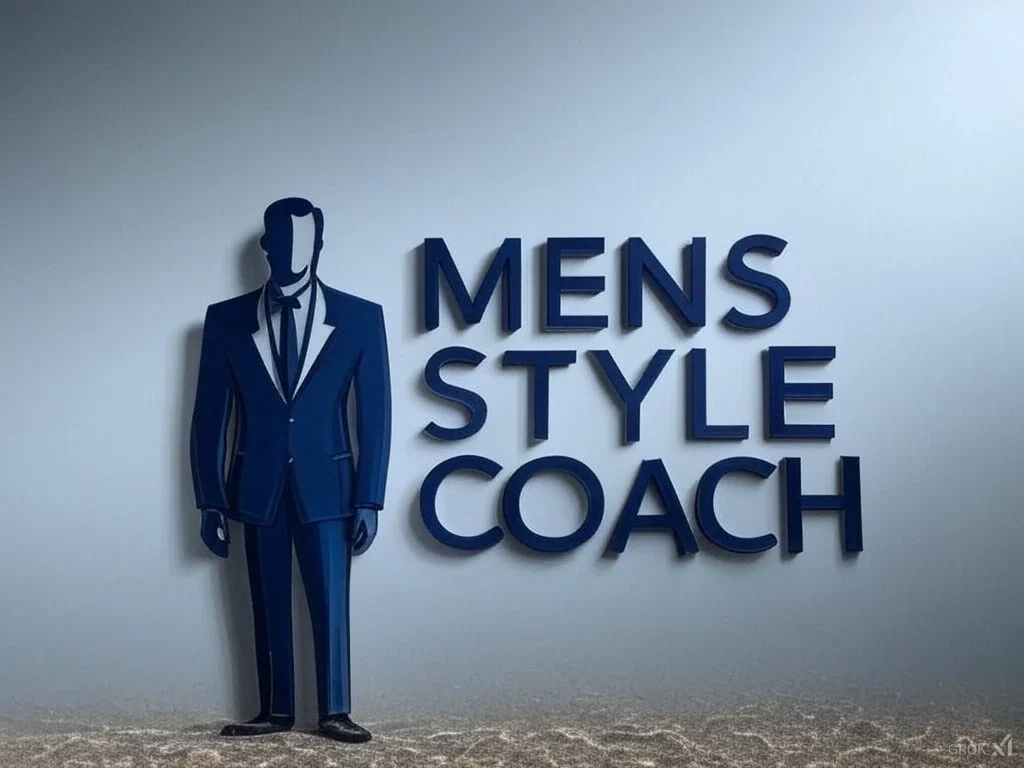
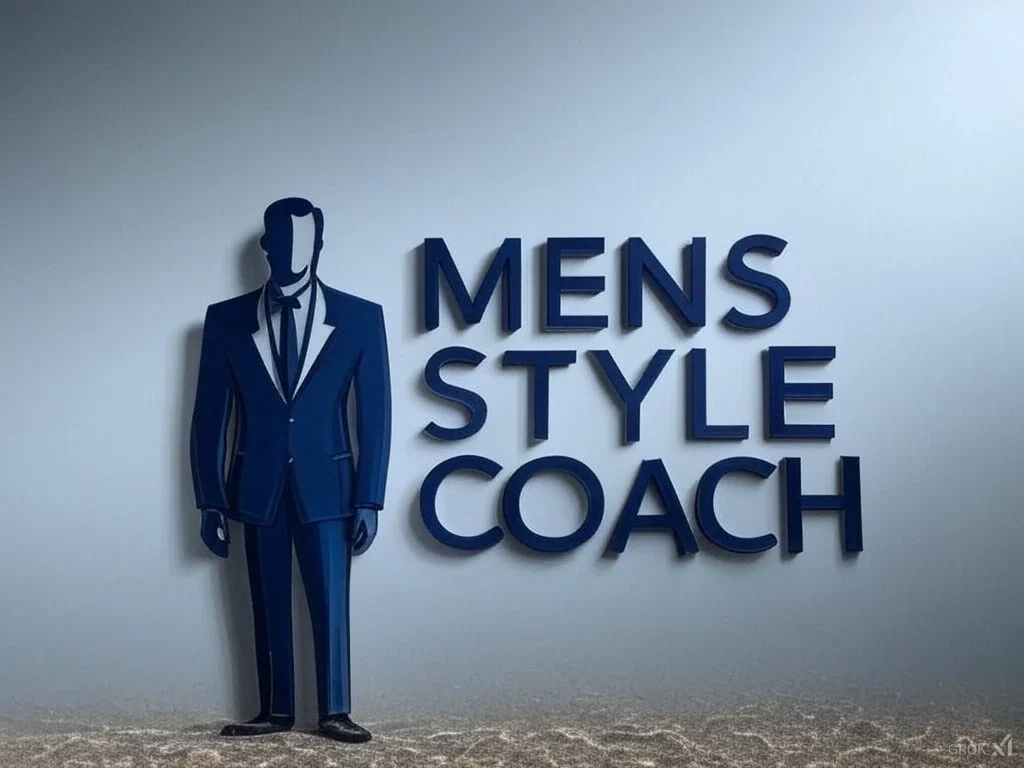
If you’re curious about specific MCP servers or want help setting one up, let me know!
specific MCP server examples
DeepSearch
35s
10 sources
Exploring MCP servers
Compiling examples
Researching additional servers
Evaluating server uses
Completed
Exploring MCP servers
- The user is asking for specific examples of MCP servers, which are part of Anthropic’s Model Context Protocol.
- I’m considering searching for concrete instances of MCP servers in use or development.
Searching for “specific MCP server examples Model Context Protocol”

Model Context Protocol (MCP) an overview
www.philschmid.de

The Model Context Protocol (MCP) — A Complete Tutorial | by Dr. Nimrita Koul | Mar, 2025 | Medium
medium.com
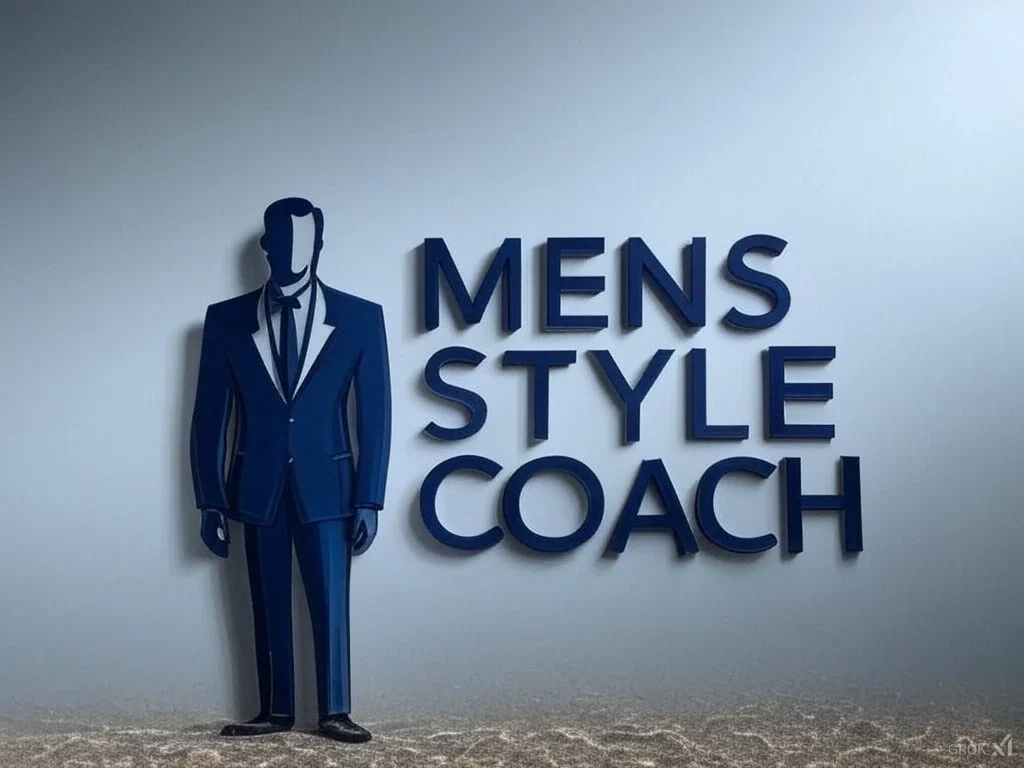
Introduction – Model Context Protocol
modelcontextprotocol.io

Model Context Protocol (MCP): A Guide With Demo Project | DataCamp
www.datacamp.com
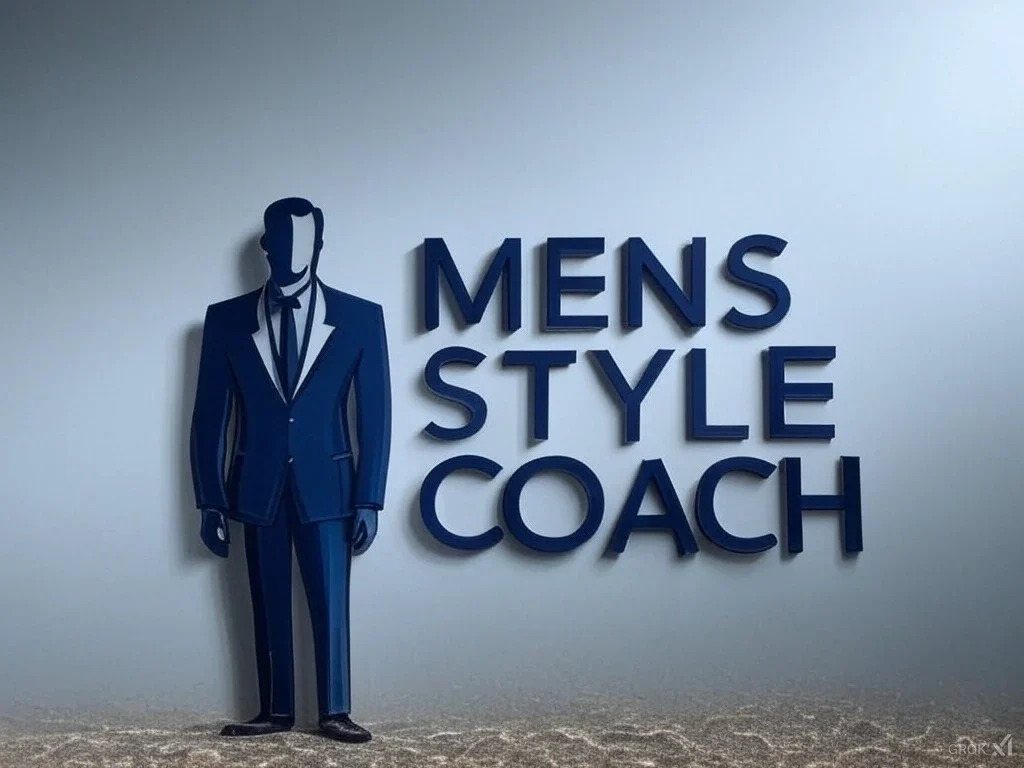
Core architecture – Model Context Protocol
modelcontextprotocol.io
See 5 more
- Just found interesting examples from the GitHub repository, like Cloudinary for media uploads and code-assistant for code changes.
- Notion integration is another example, allowing access to Notion databases (GitHub).
Browsing github.com for “specific examples of MCP servers”
- Filesystem server enables secure file operations with configurable access controls (GitHub (/modelcontextprotocol/servers/blob/main/src/filesystem)).
- Slack server supports messaging and channel management, which is quite popular (GitHub (/modelcontextprotocol/servers/blob/main/src/slack)).
- Neo4j offers a graph database server with schema and read/write capabilities (GitHub).
- There are also mentions of Azure OpenAI integration, enhancing connections with Azure services.
- I’m compiling a list, including Filesystem for local file access and GitHub for repository interactions.
- Just found more examples, like Chroma for vector search and Elasticsearch for data querying (GitHub).
- Perplexity MCP Server is useful for real-time web-wide research using Perplexity’s Sonar API.
Compiling examples
- Let’s check if there are other notable MCP servers, like those for content management or cryptocurrency data.
- Finding info on Contentful for managing website content and Crypto-feargreed for market sentiment.
Researching additional servers
- There’s a Memory MCP Server for maintaining a knowledge graph-based memory system.
- It’s great for AI needing persistent, structured data recall.
- Offers a knowledge graph-based persistent memory system, allowing AI to store and retrieve contextual information.
- Useful for maintaining long-term memory in AI applications.
Evaluating server uses
- Heroku MCP Server is useful for developers integrating AI with cloud deployment platforms.
- AI applications involving image, audio, or text similarity searches are supported by Milvus MCP Server.
Completed

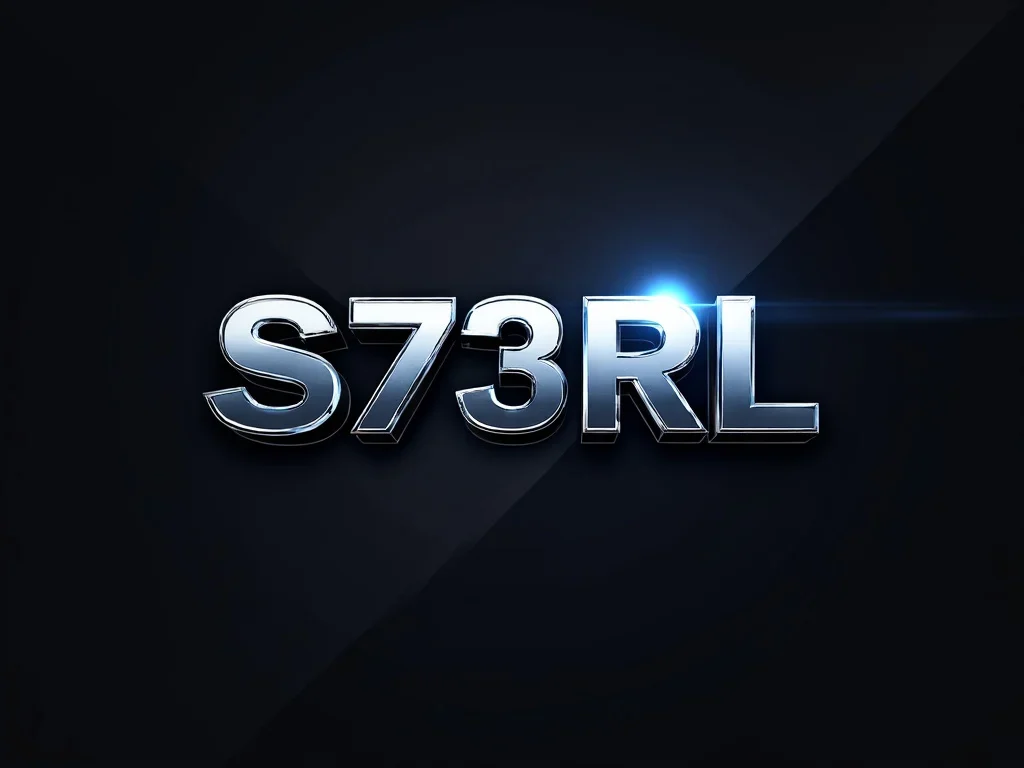




10 web pages
Key Points
- MCP servers are programs that connect AI models to external tools and data, like files or APIs.
- Examples include servers for GitHub, Notion, Slack, and Google Drive, enabling AI to interact with these services.
- Research suggests over 1,000 community-built servers exist, covering diverse tools like databases and cloud services.
What Are MCP Servers?
MCP servers, part of the Model Context Protocol (MCP) introduced by Anthropic in November 2024, are lightweight programs that act as bridges. They allow AI models, like large language models (LLMs), to connect with external data sources and tools in a standardized, secure way. Think of them as universal adapters, often called a “USB-C for AI,” enabling AI to fetch real-time data (e.g., documents, database queries) or perform actions (e.g., create a GitHub issue) without custom integrations.
Specific Examples
Here are some specific MCP server examples, showcasing their versatility:
- Filesystem MCP Server: Manages local files securely, useful for AI needing file access.
- GitHub MCP Server: Interacts with GitHub for code management, ideal for coding assistants.
- Notion MCP Server: Accesses Notion databases, helpful for productivity tools.
- Slack MCP Server: Handles Slack messaging, enhancing team communication automation.
- PostgreSQL MCP Server: Queries PostgreSQL databases, useful for data analysis.
- Google Drive MCP Server: Manages Google Drive files, great for cloud storage integration.
- Google Maps MCP Server: Provides location services, useful for navigation tasks.
- Memory MCP Server: Maintains a knowledge graph for AI memory, aiding long-term context.
- Chroma MCP Server: Offers vector search and document storage, perfect for advanced searches.
- Elasticsearch MCP Server: Queries Elasticsearch for data analysis, ideal for search-heavy applications.
These examples illustrate how MCP servers enable AI to work with a wide range of tools and services, making them more dynamic and context-aware.
Survey Note: Detailed Analysis of MCP Servers and Specific Examples
The Model Context Protocol (MCP), introduced by Anthropic in November 2024, has emerged as a significant framework for enhancing AI model interactions with external systems. This survey note provides a comprehensive overview of MCP servers, their functionalities, and specific examples, drawing from recent documentation and community contributions as of April 15, 2025. The analysis aims to offer a detailed understanding for researchers, developers, and AI practitioners, ensuring a thorough exploration of the topic.
Background and Context
MCP is an open protocol designed to standardize how AI applications, particularly large language models (LLMs), integrate with external data sources, tools, and services. It addresses the challenge of AI isolation from real-time data, unlike traditional systems like Retrieval-Augmented Generation (RAG), which rely on pre-indexed data. MCP servers, as part of this protocol, act as lightweight programs that expose specific capabilities, enabling secure and standardized connections. They are often likened to a “USB-C for AI,” providing a universal interface for AI to interact with diverse systems, such as databases, APIs, file systems, and cloud services.
The protocol follows a client-server architecture:
- MCP Hosts: AI applications like Claude Desktop or IDEs that initiate requests.
- MCP Clients: Maintain one-to-one connections with servers, acting as intermediaries.
- MCP Servers: Connect to local or remote data sources, exposing resources, tools, and prompts.
This architecture facilitates dynamic, context-aware AI interactions, reducing the need for custom integrations and enhancing scalability. As of February 2025, over 1,000 community-built MCP servers have been developed, reflecting strong adoption within Anthropic’s ecosystem and growing support across other AI platforms.
Capabilities and Benefits
MCP servers provide three main types of capabilities:
- Resources: Data sources like files, database records, or API responses, accessible without significant computation.
- Tools: Functions for performing actions, such as API calls or file operations.
- Prompts: Pre-defined templates for optimal AI interactions, selected by users before inference.
The benefits include standardization, security (credentials stay server-side with user approval often required), and scalability. This allows AI models to integrate seamlessly with enterprise tools (e.g., Jira, Confluence), development platforms (e.g., GitHub, GitLab), and daily task automation (e.g., Slack, Google Drive). However, challenges include managing multiple servers in complex setups, setup difficulties, and security concerns, particularly with sensitive data like database credentials.
Specific Examples of MCP Servers
To illustrate the versatility of MCP servers, below is a detailed list of specific examples, categorized by functionality and drawn from official documentation and community repositories, such as the Model Context Protocol Official Servers Repository. These examples cover a broad spectrum of use cases, from file management to advanced data analysis.
Category | Server Name | Description | Use Case |
---|---|---|---|
File and Storage | Filesystem | Secure file operations with configurable access controls | AI needing local file access |
Google Drive | File access and search capabilities for Google Drive | Cloud storage integration | |
Version Control | GitHub | Repository management, file operations, and GitHub API integration | Coding assistants, code management |
Git | Tools to read, search, and manipulate Git repositories | Version control automation | |
Collaboration Tools | Slack | Channel management and messaging capabilities | Team communication automation |
Notion | Access and manipulate Notion databases and pages | Productivity tool integration | |
Databases | PostgreSQL | Read-only database access with schema inspection | Data analysis and querying |
Neon | Interact with Neon’s serverless Postgres platform | Scalable database access | |
Elasticsearch | Query and analyze data in Elasticsearch | Search and analytics | |
Location Services | Google Maps | Location services, directions, and place details | Navigation and geospatial tasks |
Knowledge and Memory | Memory | Knowledge graph-based persistent memory system | Long-term context maintenance |
Chroma | Embeddings, vector search, document storage, full-text search | Advanced search and retrieval | |
Vector Databases | Milvus | Vector search and interaction with Milvus vector databases | Similarity search for images, audio |
Qdrant | Semantic memory layer on top of Qdrant vector search engine | AI memory for similarity tasks | |
Search and Analytics | Meilisearch | Full-text and semantic search using Meilisearch | Efficient text-based search |
Kagi Search | Web searches using Kagi’s search API | Privacy-focused web search | |
Cloud and DevOps | Heroku | Interact with Heroku Platform through LLM-driven tools | Cloud deployment management |
CircleCI | Enable AI Agents to fix build failures from CircleCI | CI/CD automation | |
Grafana | Search dashboards, investigate incidents, query datasources in Grafana | Monitoring and metrics analysis | |
Financial and Payments | PayPal | Integrates with PayPal’s services for payment tasks | Financial and e-commerce applications |
Research and Knowledge | Perplexity | Real-time web-wide research using Perplexity’s Sonar API | Up-to-date web-based knowledge |
This table highlights the diversity of MCP servers, covering file systems, version control, collaboration tools, databases, location services, knowledge management, vector databases, search engines, cloud and DevOps platforms, and financial services. Each server is designed to address specific needs, enhancing AI’s ability to interact with external systems.
Additional Notable Examples
Beyond the table, other notable MCP servers include:
- Cloudinary MCP Server: For uploading media to Cloudinary and retrieving media links, useful for AI-driven content management.
- Code-executor MCP Server: Allows LLMs to execute Python code within a specified Conda environment, ideal for AI programming tasks.
- Contentful MCP Server: Enables reading, updating, deleting, and publishing content in Contentful spaces, supporting content management workflows.
- Crypto-feargreed MCP Server: Provides real-time and historical Crypto Fear & Greed Index data, useful for financial AI applications.
These examples were identified from community repositories and tutorials, such as a DataCamp tutorial on building MCP servers for GitHub and Notion, which further demonstrates practical implementations.
Use Cases and Adoption
MCP servers are widely used in enterprise settings for connecting AI to internal tools like Jira or Confluence, in development for AI co-pilots managing code repositories via GitHub or Git servers, and in daily tasks for automating file management, Slack communication, or location-based tasks with Google Maps. The adoption is particularly strong within Anthropic’s ecosystem, with Claude Desktop as a key host, but broader support across platforms like Azure OpenAI and open-source LLMs is growing. As of April 2025, the community has contributed over 1,000 servers, with ongoing development reflected in SDKs for Python, TypeScript, Java, and Rust, available at modelcontextprotocol.io.
Challenges and Future Directions
While MCP servers offer significant advantages, challenges include managing multiple servers in cloud or multi-user setups, setup difficulties reported by some users, and security concerns, especially with sensitive data. The evidence leans toward MCP addressing these through standardized security practices, but ongoing research and community feedback will be crucial. Future directions may include broader platform support, improved documentation, and addressing bugs, as seen in discussions on GitHub issues for the MCP specification.
Conclusion
MCP servers represent a transformative approach to AI integration, enabling dynamic and context-aware interactions with external systems. The specific examples provided—ranging from Filesystem to Perplexity—demonstrate their versatility and utility, supporting a wide array of applications. As the ecosystem continues to grow, MCP is likely to play a pivotal role in shaping how AI interacts with the digital world, offering a standardized, secure, and scalable solution for developers and enterprises alike.
Key Citations
- Model Context Protocol Official Servers Repository
- Model Context Protocol Introduction
- Building an MCP Server for GitHub and Notion
- MCP Architecture Overview
DeepSearch
Think
Edit Image
Grok 3
beta